Modeling Climate Systems with Machine Learning Techniques
Discover how machine learning techniques are transforming climate modeling, from weather prediction to detecting anomalies, and shaping our understanding of climate change.
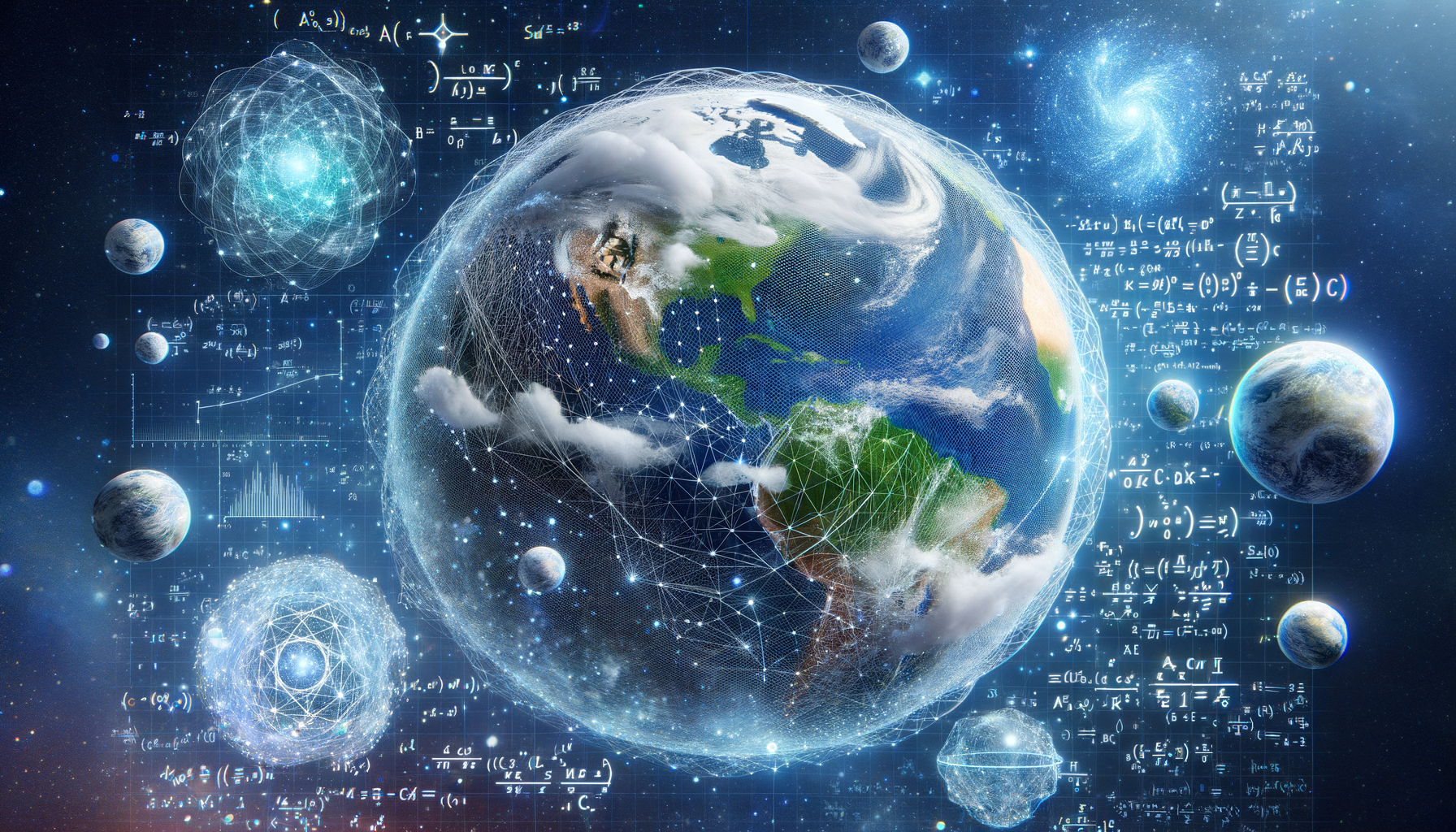
The Intersection of Climate and Technology
Have you ever wondered how scientists predict weather patterns or climate changes several decades into the future? It's mind-boggling to consider the sheer complexity of climate systems, with their countless variables and unpredictable nature. Yet, advances in technology, particularly machine learning (ML), are allowing us to make significant strides in this area.
What is Machine Learning?
Machine learning is a subset of artificial intelligence (AI) that enables computers to learn from data and improve their predictions over time without being explicitly programmed. Essentially, we feed vast amounts of data into an algorithm, which then 'learns' the underlying patterns and relationships within that data. This capability makes ML particularly useful for modeling complex systems, such as climate.
Why Use Machine Learning for Climate Models?
Traditional climate models rely on mathematical equations to simulate the interactions between the atmosphere, oceans, and land. While these models have proven to be invaluable, they can only approximate the real world so accurately. Machine learning, on the other hand, can handle vast amounts of data and uncover intricate patterns that might be missed by traditional methods. This enables the creation of more accurate and granular climate models.
Reading about how these techniques contribute to our understanding of climate is crucial for comprehending their full impact. For more detailed insights, you can check out this informative article.
Applications in Climate Modeling
- Weather Prediction: ML algorithms can predict short-term weather conditions by analyzing historical weather data and identifying patterns that precede specific weather events. This is immensely valuable for agriculture, disaster management, and daily life activities.
- Climate Change Projections: By feeding long-term climate data into ML models, researchers can gain insights into how climate variables will change over decades. This information is crucial for planning and policymaking, especially in sectors like agriculture, forest management, and urban planning.
- Anomaly Detection: ML algorithms excel at detecting anomalies. They can identify unusual weather patterns or climate events that might indicate significant changes, such as shifts in ocean currents or unusual temperature variations. These anomalies can serve as early-warning systems for impending climate crises.
Challenges and Future Directions
While the potential of ML in climate science is immense, several challenges remain. One major issue is the need for high-quality, comprehensive datasets to train the models. Additionally, integrating ML models with traditional climate models to create hybrid systems is an ongoing area of research.
Despite these challenges, the future looks promising. As computational power continues to grow and algorithms become more sophisticated, the accuracy and usefulness of ML-based climate models will only increase. It's an exhilarating time to be involved in the intersection of climate science and technology!
Conclusion
In conclusion, machine learning is revolutionizing the way we model and understand climate systems. By leveraging the capabilities of ML, we can create more accurate and comprehensive climate models that help predict weather patterns, understand climate change, and detect anomalies. Continued advancements in this field hold great promise for the future, allowing us to tackle some of the most pressing challenges facing our world today.